Soft-labels, new paper at NAACL2021!
Glad to announce that the paper
“Beyond Black & White: Leveraging Annotator Disagreement via Soft-Label Multi-Task Learning”
with Alexandra Uma, Silviu Paun, Barbara Plank, Dirk Hovy and Massimo Poesio has been accepted at NAACL2021!
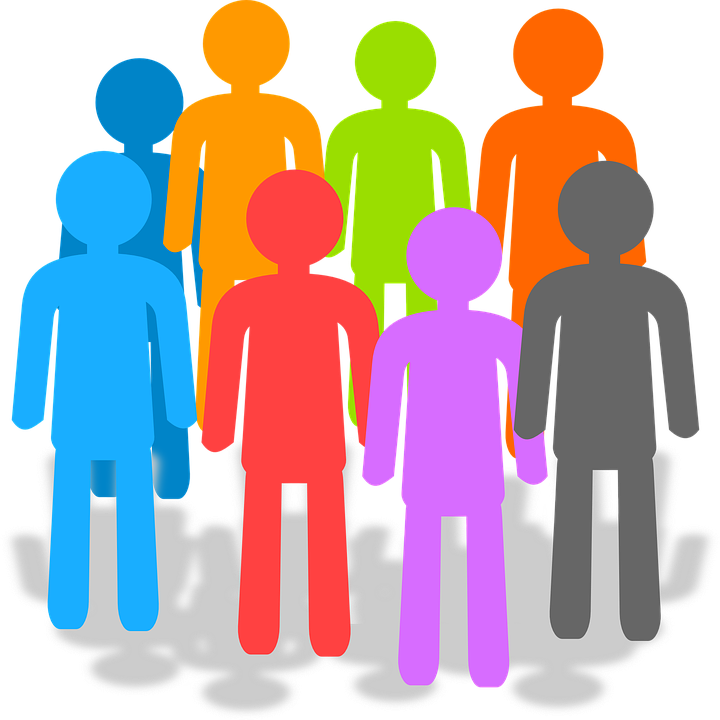
Overview
Supervised learning assumes that a ground truth label exists.
However, the reliability of this ground truth depends on human annotators, who often disagree.
Prior work has shown that this disagreement can be helpful in training models.
We propose a novel method to incorporate this disagreement as information: in addition to the standard error computation, we use soft-labels (i.e., probability distributions over the annotator labels) as an auxiliary task in a multi-task neural network.
We measure the divergence between the predictions and the target soft-labels with several loss-functions and evaluate the models on various NLP tasks.
We find that the soft-label prediction auxiliary task reduces the penalty for errors on ambiguous entities, and thereby mitigates overfitting. It significantly improves performance across tasks, beyond the standard approach and prior work.